How Nlyte Software Helps Operators Plan and Manage Their AI Footprints
Introduction
With artificial intelligence (AI) playing a central role in enterprise technology, data centers must adapt to manage this surge in demand for computing power, storage, and specialized infrastructure. AI workloads require high-density, low-latency configurations and more effective cooling, power management, and operational efficiency. As a result, traditional data center infrastructure management (DCIM) must evolve to address these needs.
Nlyte Software, a Carrier company, provides solutions tailored to the demands of AI-specific data center workloads. This article will explore how Nlyte’s DCIM tools enable data center operators to meet AI demands through effective floor planning, asset management, layout optimization, auto-allocation, power chain redundancy, and thermal management.
Floor Planning for AI Workloads
Planning the physical layout of a data center with AI workloads in mind is a critical first step in managing these infrastructures effectively. As AI workloads demand increasingly complex and resource-intensive hardware, such as high-density servers, GPUs, and specialized AI processors, traditional floor planning approaches often fall short of supporting these power- and cooling-intensive setups. The layout must account for proximity to power sources, cooling requirements, and network connectivity to avoid bottlenecks that could impact performance and increase latency. In addition, AI-specific layouts must be adaptable and scalable to accommodate rapid technology evolution and workload expansion, given that AI environments tend to grow at an accelerated pace.
Nlyte’s floor planning tools enable data center operators to strategically design and optimize floor space specifically for AI hardware. These tools assist operators in creating spatial configurations that balance energy efficiency, thermal management, and operational accessibility, all while supporting the unique needs of AI processing units. By simulating various configurations and analyzing layout scenarios, Nlyte’s floor planning solutions allow data center managers to pinpoint the optimal placement for high-performance assets, reducing the risk of heat buildup, minimizing energy waste, and supporting efficient data throughput. This AI-aware floor planning not only improves performance and sustainability but also ensures that data centers can respond swiftly to the increasing demands of artificial intelligence workloads, setting the foundation for a resilient and future-proof AI infrastructure.
Focus Capabilities
- AI-optimized Floor Space Design: Nlyte’s software provides AI-optimized floor planning solutions, allowing operators to allocate space for high-performance servers, GPUs, and specialized processors. Nlyte enables operators to simulate various configurations and determine the best layout to minimize latency and ensure that hardware resources are readily available as AI applications scale.
- Simulation and Modeling: Using Nlyte, operators can simulate AI workload impacts on the floor space layout before deployment. This enables them to evaluate heat output, cooling demands, and power consumption. Accurate simulations help prevent costly disruptions caused by inadequate space or environmental controls, especially as AI workloads grow.
- Futureproofing for AI Growth: Nlyte’s planning tools offer flexibility for scaling AI footprints over time. As AI applications require additional hardware or higher density, Nlyte makes it easier to adjust floor layouts, ensuring the data center can meet evolving AI demands without costly retrofitting.
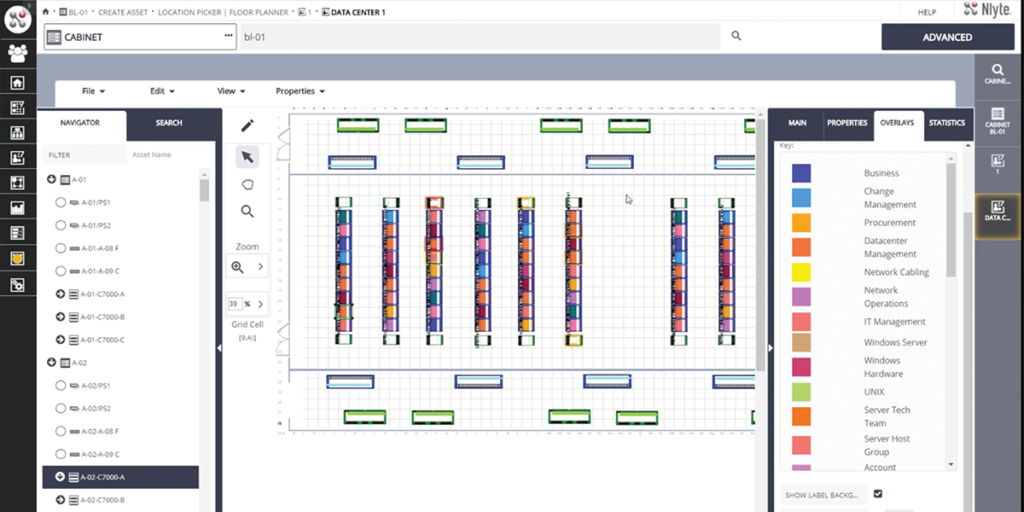
Intelligent Asset Placement and Management
Managing the placement and lifecycle of AI-dedicated hardware is essential to maintaining the high performance, cost-efficiency, and compliance standards necessary for modern data centers. Unlike traditional computing equipment, AI-specific assets—such as GPUs, TPUs, and other high-density processing units—require careful placement to optimize cooling, power, and network connectivity. These components are designed to handle intense computational tasks but come with increased energy and cooling needs, demanding specialized oversight throughout their lifecycle.
Efficient asset management for AI hardware involves more than just tracking the physical location of equipment; it requires a comprehensive view of each asset’s lifecycle, from acquisition through end-of-life decommissioning. By effectively managing this lifecycle, data centers can minimize unplanned downtime, avoid costly over-provisioning, and ensure compliance with both internal and regulatory standards. Additionally, as AI technology rapidly evolves, data centers must regularly assess and upgrade their hardware to stay competitive, making lifecycle visibility and management more critical than ever.
Nlyte’s asset management tools provide data center operators with the capability to monitor, track, and optimize AI-specific hardware across its entire lifecycle. With real-time insights into the health, utilization, and performance metrics of each asset, operators can make data-driven decisions that enhance resource allocation, reduce maintenance costs, and extend the lifespan of valuable AI infrastructure. This comprehensive approach not only ensures that AI hardware operates at peak efficiency but also supports broader data center goals around sustainability, cost management, and compliance, empowering operators to maximize the return on their AI investments.
Focus Capabilities
- Optimized Hardware Placement: AI-specific hardware, like GPUs and ASICs, requires higher power density and cooling support than typical computer assets. Nlyte’s asset management tools assist in placing these assets optimally, considering each asset’s power, space, and connectivity needs to ensure smooth operations.
- Lifecycle Management for AI Assets: From acquisition to decommissioning, Nlyte’s lifecycle management capabilities enable operators to track AI-specific assets, ensuring compliance and enhancing visibility. This helps operators forecast when assets will need replacement or upgrades, improving budget planning and minimizing the risk of downtime.
- Real-time Monitoring and Analytics: Nlyte’s real-time monitoring tools track asset performance metrics crucial for AI workloads, such as processing speed, temperature, and power consumption. Operators gain insights into asset utilization and can proactively address performance bottlenecks or maintenance needs, keeping AI applications running smoothly.
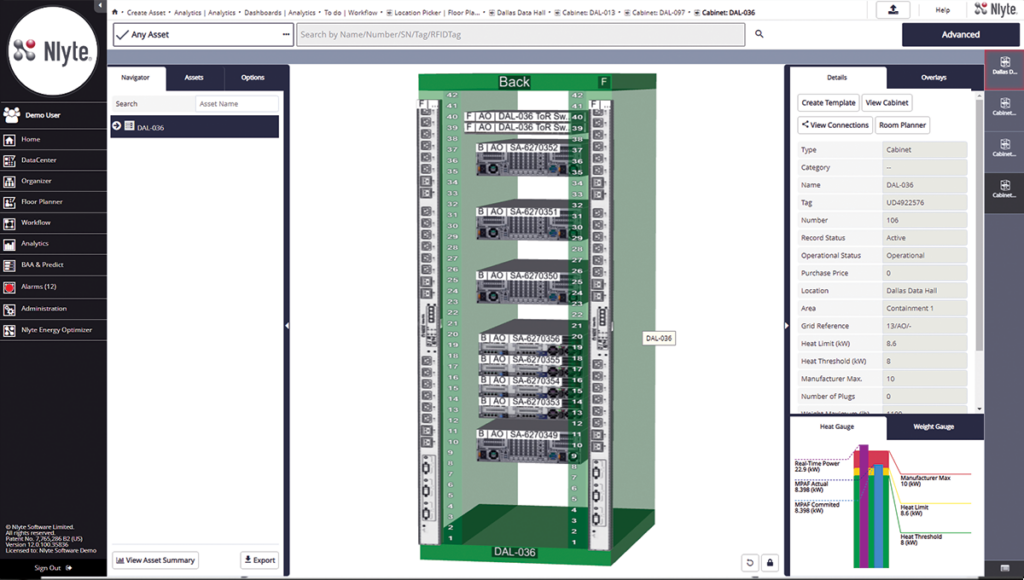
Layout Optimization for Enhanced Performance
Optimal layout design is particularly valuable for AI workloads, which come with unique demands in terms of power density, network connectivity, and cooling. Unlike traditional data center layouts, AI-focused configurations require thoughtful asset placement to reduce latency and ensure efficient thermal management, as AI hardware generates significantly more heat and relies on ultra-fast data transfer between processing units. For these high-performance environments, ensuring that AI hardware is arranged to support close-proximity networking and receive adequate, targeted cooling is essential for preventing bottlenecks and reducing operational costs.
Nlyte’s layout optimization tools are purpose-built to address these challenges, allowing data center operators to design and organize AI asset layouts that maximize both performance and resource utilization. By analyzing power and cooling requirements alongside spatial constraints, Nlyte’s tools assist in configuring hardware placement to create optimal “hot” and “cold” zones, reduce signal degradation in high-speed data paths, and minimize physical and network latency between assets. This kind of strategic layout optimization not only bolsters system performance but also improves energy efficiency, as resources are allocated precisely where they’re needed.
Furthermore, Nlyte’s layout optimization enables data centers to adapt quickly to the evolving nature of AI workloads. As new hardware with higher performance requirements is deployed, operators can easily adjust layouts to integrate these assets without sacrificing efficiency or uptime. By supporting a modular, flexible approach to layout design, Nlyte empowers data centers to stay agile and resilient in a landscape where AI demands continue to grow, ensuring that they can meet both current needs and future scalability requirements.
Focus Capabilities
- Layout Optimization Algorithms: Nlyte’s software employs layout optimization algorithms that consider power, cooling, and connectivity demands to ensure that AI workloads are supported in a way that minimizes latency and maximizes efficiency. This is especially important for the high data transfer needs common with AI applications.
- Efficient Cable and Connectivity Planning: For AI, low latency is crucial. Nlyte supports operators in planning optimal cable pathways, reducing signal loss and minimizing the physical distance between high-performance AI hardware to ensure rapid data processing.
- Dynamic Layout Adjustments: Nlyte makes it easy for operators to adjust their layouts as AI workload demands shift. These adjustments can be done with minimal downtime, allowing data centers to respond quickly to changes in AI application needs without sacrificing productivity.
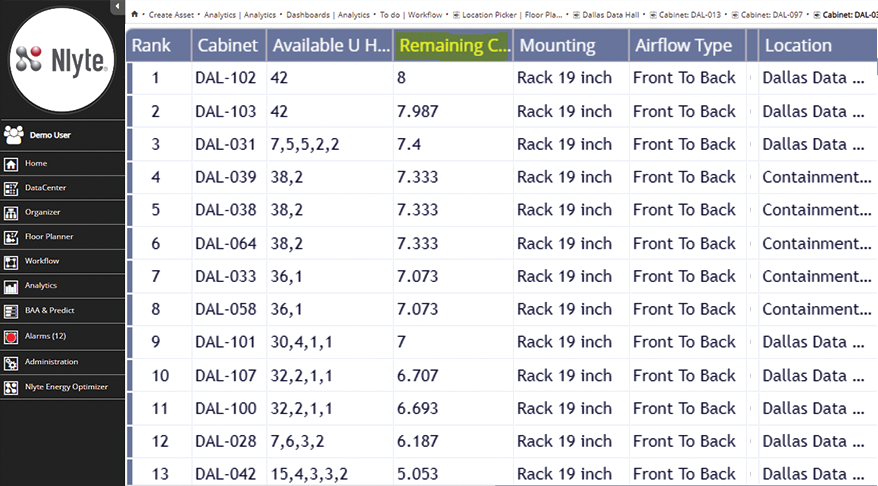
Auto-allocation of Resources for AI
Automated allocation of resources is a valuable feature for data centers managing the dynamic and often unpredictable demands of AI workloads. AI applications are highly data-intensive, requiring large amounts of server, memory, and storage resources that must be provisioned quickly and efficiently to avoid disruptions. Manual resource allocation can be time-consuming and prone to errors, leading to delays in scaling up for new AI tasks or in reallocating resources when demand shifts. In the context of AI, where workloads can spike unpredictably or require rapid scalability, automated allocation becomes essential to maintaining optimal performance and minimizing operational overhead.
Nlyte’s auto-allocation capabilities simplify the process of provisioning, reallocating, and scaling resources, providing a seamless, hands-free approach that ensures AI applications have access to the resources they need, precisely when they need them. This automation reduces the dependency on manual intervention, which not only speeds up deployment times but also minimizes the risk of human error and resource wastage. By dynamically responding to real-time demands, Nlyte’s auto-allocation tools enable data centers to maximize the efficiency of their hardware and avoid costly over-provisioning or resource shortages.
In addition, Nlyte’s automated resource allocation integrates with broader capacity planning, allowing data center operators to anticipate future resource needs based on AI workload patterns. This proactive approach ensures that data centers are prepared to scale quickly, supporting smooth operations even as AI workloads increase in complexity and volume. As a result, Nlyte’s auto-allocation functionality not only enhances operational efficiency but also provides data centers with a robust framework for growth and adaptability in the face of evolving AI demands.
Focus Capabilities
- Automated Resource Provisioning: With Nlyte’s auto-allocation, data centers can streamline the process of assigning resources to AI applications. By automatically provisioning resources as needed, Nlyte reduces setup complexity and speeds up deployment times, allowing for faster scaling and responsiveness.
- Demand-based Scaling for Efficiency: Nlyte’s system can allocate resources dynamically based on real-time AI workload demands, which helps prevent both under- and over-provisioning. This ensures that resources are used efficiently, optimizing energy usage and reducing costs.
- Integrated Capacity Planning: The capacity planning tools within Nlyte help operators predict future resource demands based on AI application trends. This proactive approach to resource management ensures that operators are prepared for increasing AI workloads and can scale resources appropriately.
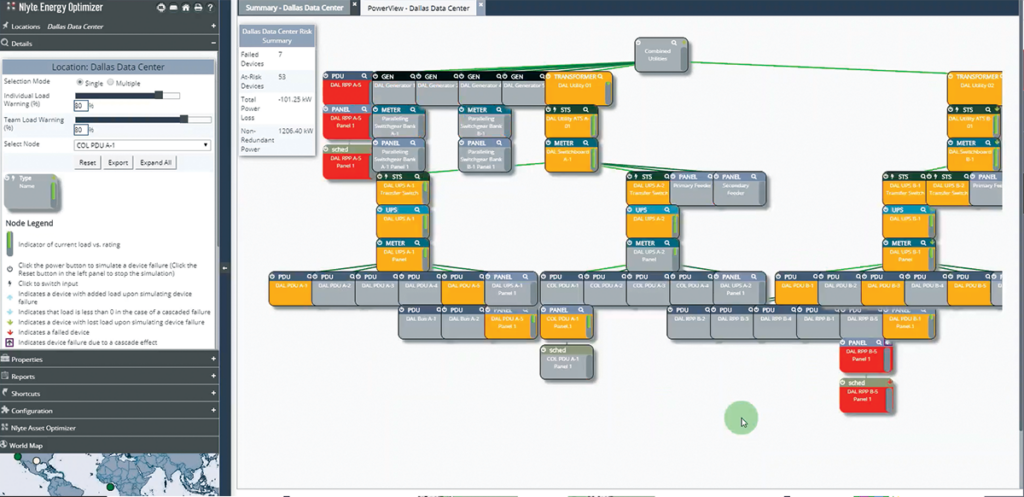
Power Chain Redundancy Planning to Ensure Reliability
Maintaining reliable power is essential for AI workloads, as they are highly sensitive to disruptions and require continuous, stable power to sustain performance and prevent data loss. AI applications, which run on dense, high-performance hardware, rely on uninterrupted power to avoid setbacks like halted data processing or hardware damage—issues that can arise even from minor power fluctuations. Given the scale of investment in AI infrastructure and the critical nature of AI-driven insights, safeguarding power continuity is a top priority for data center operators.
Nlyte Software’s PowerView power chain planning tool provides data centers with the comprehensive resources needed to secure power continuity for AI workloads. PowerView enables data center operators to design power chain redundancy with multiple layers of backup, creating robust, fail-safe power pathways that activate instantly in case of primary power interruptions. With PowerView, data centers can implement seamless, automated power switching and failover, ensuring AI applications continue running without disruption.
Beyond redundancy, PowerView’s advanced monitoring and predictive maintenance capabilities offer operators real-time insights into the health of the entire power chain. The tool’s automated alerting system detects potential issues early on, allowing for timely intervention and reducing the risk of unexpected power failures. PowerView’s analytics on power usage trends and infrastructure wear-and-tear also support proactive management strategies, helping operators optimize power distribution and reduce energy costs.
By integrating these layers of redundancy, monitoring, and maintenance, PowerView enables data centers to achieve high uptime and resilience while meeting cost-efficiency and sustainability goals. PowerView equips data centers with the tools they need to confidently meet AI workload demands, ensuring reliable, continuous power to support both current and future AI initiatives.
Focus Capabilities
- Redundant Power Systems: AI workloads require high uptime, and Nlyte’s power chain redundancy planning tools help to reduce risks associated with power outages. In the event of a primary power failure, redundant systems can keep critical AI operations running smoothly.
- Automated Alerts and Predictive Maintenance: Nlyte’s software monitors power infrastructure for potential issues and provides automated alerts and predictive maintenance recommendations. Early identification of issues reduces the chance of unexpected downtime, allowing operators to resolve power concerns before they impact AI workloads.
- Energy Efficiency and Sustainability Initiatives: With power optimization tools, Nlyte enables data centers to balance AI workload demands with energy efficiency and sustainability goals. This is increasingly important as data centers seek to reduce their environmental footprint while supporting AI growth.
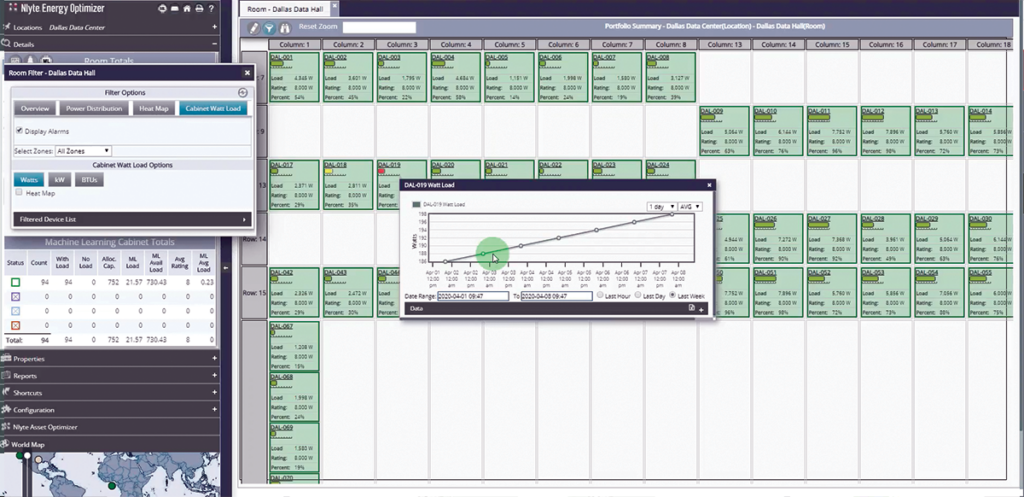
Thermal Management for High-density AI Hardware
AI workloads require high-density, high-performance hardware such as GPUs and TPUs, which generate intense levels of heat due to their processing demands. Without effective thermal management, this heat can lead to performance degradation, reduce the lifespan of assets, and increase operational costs due to higher energy consumption. As a result, efficient cooling becomes a cornerstone of AI infrastructure management, ensuring that AI-specific hardware operates reliably and at peak performance. Managing the thermal demands of these systems is not only essential for asset protection but also for driving energy efficiency—a key consideration as data centers strive to reduce their environmental footprint.
Nlyte’s thermal management tools provide data centers with a comprehensive approach to cooling management, specifically designed to meet the demands of high-density AI hardware. These tools enable data center operators to optimize cooling delivery by aligning it precisely with AI workload demands. By identifying areas of highest heat output and establishing cooling zones tailored for AI, Nlyte’s thermal management solutions allow data centers to reduce unnecessary cooling in low-demand areas, lower energy consumption, and enhance sustainability.
Focus Capabilities
- AI-specific Cooling Zones: Nlyte helps data center operators design and manage cooling zones that cater specifically to high-density AI hardware, grouping assets with similar thermal requirements into targeted zones. This segmentation enables more efficient resource allocation, directing cooling power where it’s needed most and reducing unnecessary energy use. By grouping high-heat-output assets in dedicated zones, data centers can minimize thermal bleed between different equipment types, leading to lower overall cooling costs and improved thermal efficiency.
- Real-time Temperature Monitoring and Dynamic Response: Using a network of sensors and advanced analytics, Nlyte enables data centers to monitor temperatures in real-time across all zones, instantly identifying any areas where overheating risks may arise. These real-time insights allow operators to proactively adjust cooling levels in response to AI processing demands, preventing overheating and ensuring hardware protection. Nlyte’s dynamic response capability means that data centers can fine-tune cooling on the fly, making real-time adjustments as workload intensity fluctuates. This not only protects critical AI infrastructure but also ensures that cooling resources are used optimally, preventing excessive energy consumption.
- Proactive Heat Management for Longevity and Performance Stability: By integrating proactive heat management into data center operations, Nlyte helps operators maintain stable temperatures, which is crucial for the longevity and performance of high-density AI hardware. Stable temperatures minimize wear on components, reducing the need for frequent repairs or replacements and lowering the risk of sudden equipment failure. This approach translates into significant cost savings on maintenance and asset replacement over time, enhancing the overall ROI on AI infrastructure investments.
- Sustainability and Cost Savings through Optimized Cooling Efficiency: Efficient thermal management doesn’t just protect assets; it also reduces energy consumption, supporting sustainability goals and driving down operational costs. By optimizing cooling delivery and avoiding overuse, Nlyte helps data centers align with environmental targets, reducing their carbon footprint and contributing to long-term sustainability. This efficiency-driven approach allows data centers to support growing AI workloads in a cost-effective, eco-friendly manner, balancing performance demands with responsible energy use.
Conclusion
As AI technology becomes increasingly integral to enterprise functions, data centers face the task of adapting to handle these unique, high-performance workloads. Nlyte Software, a Carrier company, offers data center operators a suite of tools designed to manage the specific demands of AI applications, from floor planning and asset management to power chain redundancy and thermal optimization.
By leveraging Nlyte’s comprehensive DCIM platform, data centers can efficiently scale AI workloads while maintaining high performance, minimizing downtime, and supporting sustainability goals. Nlyte empowers data centers to stay agile and responsive to the evolving landscape of AI, ensuring that operators can meet present and future demands.
Glossary of Terms Used Defined In the context of an AI Data Center
AI workloads refer to the computational tasks and processes associated with various artificial intelligence applications and models. These workloads can be broadly categorized into three main types:
- Training: This involves building and optimizing AI models by feeding them large volumes of data and adjusting their parameters through iterative learning algorithms. Training is highly computationally intensive and requires significant processing power, memory, and storage.
- Fine-Tuning: This process further optimizes a pre-trained AI model for a specific task or dataset. Fine-tuning starts with a model that has already been trained on a large dataset and adjusts its parameters to better fit a more specific dataset. It is less resource-intensive than full-scale training but still requires substantial computational resources1.
- Inference: Inference involves using trained AI models to make predictions, classifications, or decisions based on new input data. While generally less resource-intensive than training, inference still demands significant computational power, especially for real-time or low-latency applications
DCIM (Data Center Infrastructure Management) refers to a suite of tools and practices designed to manage and optimize the physical infrastructure of a data center. DCIM solutions integrate IT and facility management disciplines to provide a holistic view of a data center’s performance.
Dynamic Response refers to the ability of the data center infrastructure to adapt and respond in real-time to changing workloads and environmental conditions. This capability is crucial for maintaining optimal performance, efficiency, and reliability in environments where computational demands can fluctuate rapidly due to AI and machine learning tasks.
GPUs (Graphics Processing Units) are specialized hardware designed to accelerate the processing of large amounts of data, particularly for tasks involving complex computations and parallel processing. Unlike traditional CPUs (Central Processing Units), which are optimized for sequential processing, GPUs excel at handling multiple operations simultaneously, making them ideal for AI and machine learning workloads.
High-density AI Hardware refers to the deployment of a large amount of computing power within a compact physical space. This setup is designed to maximize efficiency and performance, particularly for AI and machine learning workloads that require significant computational resources.
Low-latency Configurations refer to the setup and optimization of hardware and network infrastructure to minimize delays in data processing and transmission. Low latency is crucial for AI applications that require real-time or near-real-time responses, such as autonomous systems, high-frequency trading, and interactive AI services.
PowerView from Nlyte Software is a component of their Data Center Infrastructure Management (DCIM) suite. PowerView provides detailed monitoring and management of power usage within the data center. It helps data center operators optimize energy consumption, improve efficiency, and reduce costs by offering real-time visibility into power usage at various levels, from individual devices to entire facilities.
Sustainability Goals refer to the specific objectives and targets set to minimize the environmental impact of data center operations. These goals aim to enhance energy efficiency, reduce carbon emissions, and promote the use of renewable resources, ensuring that the data center operates in an environmentally responsible manner. More on this topic: Fundamental Measures of Data Center Sustainability eBook
Thermal Management refers to the strategies and technologies used to control the temperature of computing hardware and the surrounding environment. Effective thermal management is crucial for maintaining the performance, reliability, and longevity of the data center’s equipment, especially given the high heat output from AI and machine learning workloads.
TPUs (Tensor Processing Units) are specialized hardware accelerators designed by Google specifically for machine learning tasks. TPUs are optimized for the high computational demands of training and running deep learning models, particularly those built using TensorFlow, Google’s open-source machine learning framework.